Gait Analysis
Movement of the human body is achieved through a complex interaction of muscles, skeleton and the central nervous system. Possible impairments of one of these anatomical systems can be detected at an early stage by experts using a gait analysis. For this purpose, for example, step length, walking speed, step frequency, joint angle, forces acting on the joints, muscle activity and energy consumption are evaluated. This information can be recorded using sensors.
In cooperation with Prof. Kerstin Lüdtke (Physiotherapy, University of Lübeck) and the company Future-Shape GmbH, we are developing artificial intelligence methods to automate and objectify gait and movement analysis. To record the necessary data, we use the SensFloor, RGB cameras, depth sensors and wearables (IMUs), among other things. The SensFloor is a sensor that can be laid widely under normal flooring and measures the electrical capacities of people and objects on it. Using this information, we can then calculate the geometric and temporal information of movement patterns. IMUs are usually attached directly to the body and record data such as acceleration, rotation rate and orientation of the test participants. In addition, information such as joint angles and their positions can be extracted from the camera images. We use all this data to differentiate between pathological and healthy gait patterns, as well as to define a general movement behaviour.
From an AI methodology perspective, we use conventional Deep Learning techniques in this context, as well as algorithms we have developed ourselves that compute the spatiotemporal motion features of human gait for individual identification, without the need for traditional silhouette extraction from video sequences. For example, the extracted local motion data is coded into high-level descriptors using the so-called codebook approach based on the Gaussian mixture model and classified using a simple linear Support Vector Machine. We have already tested our pattern recognition platform on five widely used gait databases and obtained a recognition rate of up to 98%. Another approach deals with person identification and event recognition using motion data recorded by the SensFloor. In this area, we are working on real-time processing of SensFloor data using LSTM and unsupervised learning algorithms.
Contact person
Dr.-Ing. Szymon Sieciński
Publications
Khan, Muhammad Hassan and Farid, Muhammad Shahid and Grzegorzek, Marcin. A Non-linear View Transformations Model for Cross-view Gait Recognition. Elsevier Neurocomputing, April 2020.
Khan, Muhammad Hassan, Muhammad Shahid Farid, and Marcin Grzegorzek. Spatiotemporal features of human motion for gait recognition. Signal, Image and Video Processing 13.2 (2019): 369-377.
Liebenow, Laura and Walter, Jasmin and Hoffmann, Raoul and Steinhage, Axel and Grzegorzek, Marcin.
Classifying Changes in Motion Behaviour Due to a Hospital Stay Using Floor Sensor Data--A Single Case Study. International Conference on Information Technologies in Biomedicine, Springer 2022
Bader, Felicia and Liebenow, Laura and Steinhage, Axel.
Using Deep Learning to Identify Persons by their Movement on a Sensor Floor. Proceedings of the 8th international Workshop on Sensor-Based Activity Recognition and Artificial Intelligence, 2023
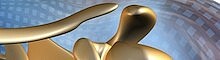
- Research
- AI und Deep Learning in Medicine
- Medical Image Processing and VR-Simulation
- Integration and Utilisation of Medical Data
- Sensor Data Analysis for Assistive Health Technologies
- Medical Image Computing and Artificial Intelligence
- Medical Data Science Lab
- Medical Deep Learning Lab
- Junior Research Group Diagnostics and Research of Movement Disorders
- Former Medical Data Engineering Lab
Contact person
Szymon Sieciński
Research Assistant
Gebäude 64, 2nd floor
,
Raum 09
szymon.siecinski(at)uni-luebeck.de