Assessment of General Movements
General Movements are spontaneous movement patterns in infants aged 3 to 5 months. A specific type of General Movements extensively studied in the literature are Fidgety Movements, that designate subtle, multidirectional and low amplitude movements observed in all parts of the body (i.e. neck, limbs and trunk). Abnormal, missing or sporadic fidgety movements are associated with a high risk of later neurological dysfunction (especially cerebral palsy). On the other hand, the occurrence of normal fidgety movement patterns is highly likely to indicate an inconspicuous neurological development in children. For these reasons, the detection and classification of this type of movements in infants is extremely important so that appropriate intervention can be initiated at an early stage. This screening process is usually performed by clinicians in the frame of the Prechtl’s General Movement Assessment procedure. However, the latter is not automatically carried out for all infants due to it being time consuming and requiring specifically trained medical personnel. For these reasons, automating the detection of Fidgety Movements is a goal of high interest for the medical community.
In close cooperation with Prof. Ute Thyen (Head of the Neuropaediatrics and Social Pediatrics Center, University Medical Center Schleswig-Holstein in Lübeck), we are developing a learning-based pattern recognition platform that will automate the General Movements examination using algorithms. As data input, we use images from two RGBD (i.e. colour and depth) cameras (Microsoft Azure and iPhone 12 Pro) and four Inertial Measurement Units (IMUs) sensors (Suunto Movesense IMU) placed on the shoulders and the hips of the infants. Following the standard methodology of pattern recognition, the classification algorithms of the platform are trained with sensor data, which are manually evaluated by the medical experts of our consortium specifically trained to detect fidgety movements. The results of the automated recognition and classification of movement patterns will be presented to the treating physicians on site using an app and, if necessary, transferred telemedically to a specialized medical facility. Another important goal of ours is also to significantly improve the availability of this examination so that it can be made available nationwide (even in rural areas).
Methodologically, we are working on three pattern recognition approaches for the detection of Fidgety Movements in this context. Firstly, we investigate the use of classification methods based on manually selected simple statistical features. Secondly, we develop a hierarchical recognition algorithm that first performs the classification separately along the assessment criteria used by medical experts (e.g. complexity, variation, fluency) and only finally fuses the results. Finally, we implement deep learning algorithms to automatically learn features relevant to the recognition of fidgety movements.
Contact person
Dr.-Ing. Frédéric Li
Third party funded projects
BMBF Project: ScreenFM - Sensor-based Assessment of Infants' Neurological Development Based on Fidgety Movements (grant number: 13GW0444E). Duration: 01.05.2021 - 30.04.2024.
Publications
M. T. Irshad, M. A. Nisar, P. Gouverneur, M. Rapp, M. Grzegorzek, AI approaches towards Prechtl's assessment of general movements: a systematic literature review, Sensors (MDPI), Vol. 20, No. 18, 2020.
F. Lentzsch, F. Li, F. Pagel, M. Lau, K. Otte, H. M. Röhling, A. Stein, A. Nisar, L. Zieser, S. Glende, N. Kaartinen, S. Mansow-Model, U. Thyen, M. Grzegorzek, Deep feature learning for fidgety movement detection using inertial measurement unit data, Student Conference Proceedings 2023 (Infinite Science Publishing), 2023
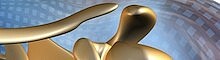
- Research
- AI und Deep Learning in Medicine
- Medical Image Processing and VR-Simulation
- Integration and Utilisation of Medical Data
- Sensor Data Analysis for Assistive Health Technologies
- Medical Image Computing and Artificial Intelligence
- Medical Data Science Lab
- Medical Deep Learning Lab
- Junior Research Group Diagnostics and Research of Movement Disorders
- Former Medical Data Engineering Lab
Contact person
Frédéric Li
Senior Reseacher
Gebäude 64, 2.OG
,
Raum 04
frederic.li(at)dfki.de
+49 451 3101 5613