Sleep Analysis
We spend about one third of our life time sleeping. Sleep, as a necessary process for sustaining life, is an important part of the recovery, integration and consolidation of the body's memory. Poor quality of sleep can in the long run lead to inattention, personality disorders and depression, weakened immunity and other symptoms. However, sleep disorders are not only a problem for adults, they also occur in children. Several studies have shown that the prevalence of sleep disorders in children is even significantly higher than in adults. Therefore, sleep stage analysis is urgently needed, especially in infants and children.
In cooperation with the DRK-Kinderklinik Siegen gGmbH we are developing a learning-based pattern recognition platform for the automatic analysis of time series from sensor data in order to optimize the classification of sleep into sleep stages. In the sleep laboratory we collect polysomnographic recordings of different sensors such as EEG, EOG and EMG. For this purpose electrodes are placed on the patient's head. Based on this data, our pattern recognition software can determine the sleep phases and diagnose possible sleep disorders in infants and children at an early stage.
In addition to classification procedures based on manually defined features, we rely on complex neural networks in this context. A major advantage of deep learning algorithms is that the raw data from sleep lab sensors can be used without the intermediate step of manual feature definition. Specifically, we are testing different approaches from the field of Deep Neural Networks, where the so-called DeConvolutional Neural Network currently achieves the best results and detects sleep stages in infants and children with a classification rate of about 85%. In addition, an attention-based model called the DeConvolution- and Self-Attention-based Model is tested to increase the explainability of the behavior of DNNs and significantly improve the detection accuracy of the sleep transition stage. It can provide strong performance in sleep stage classification with an overall accuracy of 91%.
A contrastive learning model based on data augmentation was also proposed for real-world clinical use, which provides stable and efficient classification performance for sleep stages (accuracy of 92%) and accelerates the model building and implementation process by using a meta-learning strategy with a novel truncated cross-entropy loss function across different datasets. In particular, the detection sensitivity of the sleep transition stage can reach about 75%.
Contact person
Dr.-Ing. Xinyu Huang
Publications
X. Huang, K. Shirahama, F. Li, and M. Grzegorzek. Sleep Stage Classification for Child Patients Using DeConvolutional Neural Network. Artificial Intelligence in Medicine (Elsevier, IF: 5.326), 2020, Vol.110, pp. 101981.
X. Huang, K. Shirahama, M. T. Irshad, M. A. Nisar, A. Piet, and M. Grzegorzek. Sleep Stage Classification in Children Using Self-Attention and Gaussian Noise Data Augmentation. Sensors (MDPI, IF: 3.9), 2023, 23(7), 3446.
X. Huang, F. Schmelter, M. T. Irshad, A. Piet, M. A. Nisar, C. Sina, and M. Grzegorzek. Optimizing sleep staging on multimodal time series: Leveraging borderline synthetic minority oversampling technique and supervised convolutional contrastive learning. Computers in Biology and Medicine (Elsevier, IF: 7.7), 2023, 107501.
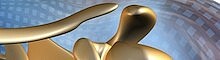
- Research
- AI und Deep Learning in Medicine
- Medical Image Processing and VR-Simulation
- Integration and Utilisation of Medical Data
- Sensor Data Analysis for Assistive Health Technologies
- Medical Image Computing and Artificial Intelligence
- Medical Data Science Lab
- Medical Deep Learning Lab
- Junior Research Group Diagnostics and Research of Movement Disorders
- Former Medical Data Engineering Lab