CoCoAI: Cooperative and Communicating AI methods for medical image-guided diagnostics (BMBF)
Artificial intelligence (AI), which can learn from medical expertise, will play an important role in the health care system and enable fundamental improvements in the accuracy and efficiency of image-based medical diagnosis and treatment.
CoCoAI's goal is the evidence-based development of information and communication technologies that best support collaboration between AI and humans. Future applications of AI in healthcare will not only improve diagnostic quality, but also influence many ethical, legal, social and economic aspects of modern medicine. We are working towards overcoming the ethical and social challenges that arise from the integration of AI into existing structures of action and will be devising methods that will positively support the new role perception of the three main actors (patients, doctors and AI engineers). We will create methodological foundations for trilateral cooperation and communication through explainable AI methods, modern human-computer interfaces as well as ethical studies and workshops to define responsible research and innovation with AI. In the research group of Prof. Heinrich new strategies for the efficient and user-friendly visualisation of deep learning results will be developed and implemented, e.g. by limiting them to simple geometric shapes and virtual 3D views.
Furthermore, in the course of the project we will develop guidelines for the design of future AI- and image-based diagnostic systems from the interdisciplinary perspectives of machine learning, medical image analysis, user-centered design of human AI interfaces, visualisation and technology ethics. These guidelines will promote the acceptance of AI-supported diagnostics, enable the realisation of new potentials and reduce risks. Together with the SME ThinkSono, we are planning a case study for the practical implementation of an ultrasound-based diagnostic system for the detection of deep vein thrombosis, the most frequent cause of avoidable death in hospitals.
BMBF project funding (2020-2024) 874’615€ UzL (ca 368'850€ IMI)
Selected Publications
- Hansen L., Heinrich M.P.
Sparse Structured Prediction for Semantic Edge Detection in Medical Images.
In: International Conference on Medical Imaging with Deep Learning. 2019. Proceedings of Machine Learning Research Vol. 102 S. 250-259. - Tanno R., Makropoulos A., Arslan S., Oktay O., Mischkewitz S., Al-Noor F., ... & Heinrich M.P.
AutoDVT: Joint real-time classification for vein compressibility analysis in deep vein thrombosis ultrasound diagnostics.
In: International Conference on Medical Image Computing and Computer-Assisted Intervention 2018 (pp. 905-912). Springer, Cham.
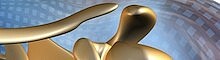
- Research
- AI und Deep Learning in Medicine
- Medical Image Processing and VR-Simulation
- Integration and Utilisation of Medical Data
- Sensor Data Analysis for Assistive Health Technologies
- Medical Image Computing and Artificial Intelligence
- Medical Data Science Lab
- Medical Deep Learning Lab
- Junior Research Group Diagnostics and Research of Movement Disorders
- Former Medical Data Engineering Lab