MEIDIC-VTACH Collaborative Project: Mobile Electrocardiographic Imaging for the Prediction of Ventricular Tachycardia (BMBF, ERA-CVD)
Patients suffering from heart failure are at risk of developing ventricular tachycardia. Ventricular tachycardia is responsible for about 80% of sudden cardiac deaths. Therefore, there is a great need to develop a better stratification of patients at risk for ventricular tachycardia.
With this goal in mind, electrocardiographic imaging (ECGI) has recently been explored to enable fusion of imaging and electrophysiological modalities for non-invasive visualization of cardiac electrophysiology.
The main purpose of this project is to develop a novel technique, the mobile ECGI. This enables the merging of electrocardiogram (ECG) data, mechanical information of the heart recorded with magnetic resonance imaging (MRI) and rhythm information from portable long-term ECG systems. To achieve this goal, three project partners from France, Israel and Germany are striving to accomplish:
- The development of a new acquisition strategy for cardiac MRI imaging of pathological rhythms. This will provide mechanical information of cardiac contraction during pathological heart beats (INSERM, IADI; Nancy France, project coordination)
- Exploring novel methods for segmentation and surface tracking of 3D+time geometries that will be integrated into the ECGI reconstruction problem (University of Lübeck, IMI; Germany)
- Implementing algorithms for automated analysis of long-term ECG signals from recording in home environment. These long-term recordings will detect rare pathological ventricular heartbeats and thus improve risk stratification (Technion Institute, AIMLab; Haifa, Israel)
In the team of Prof. Heinrich the following aspects are considered in particular:
- A precise segmentation of cardiac geometry that is robust to subtle image variations in MRI acquisitions, noise and acquisition artifacts due to patient motion
- The transformation of cardiac geometry of inter-individual MRI images into a common image space through deformable image registration, design and training of Convolutional Neural Networks (CNNs), and learning-based methods for image registration
- Reducing uncertainty in segmentation by combining deep label fusion and online learning approaches incorporating concepts on deformable convolutions, attention gates, and shape models of cardiac anatomical geometry
- The transformation of dense voxel data to a low-dimensional surface and the development of a motion tracking algorithm with graph convolutional networks to estimate patient-specific 3D+time surface models of the beating heart
- Extracting 3D+time anatomical correspondences of a patient population for statistical and morphological comparison of arrhythmic motion patterns
- Performing a proof-of-concept with project partners to demonstrate clinical potential and ability to combine and integrate work products
- The ongoing integration of the software components into the French consortium leader's ArchiMed system to automate the algorithms for future applications
BMBF, ERA-CVD project 01KL2008, funding (2020-2023) 264'017€ (IMI)
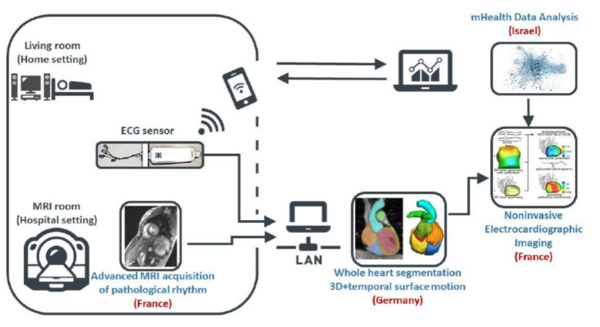
Selected Publications
Hering, A., Kuckertz, S., Heldmann, S., & Heinrich, M. P. (2019). Enhancing Label-Driven Deep Deformable Image Registration with Local Distance Metrics for State-of-the-Art Cardiac Motion Tracking. In Bildverarbeitung für die Medizin 2019 (pp. 309-314). Springer Vieweg, Wiesbaden.
Schlemper, J., Oktay, O., Schaap, M., Heinrich, et al. (2019). Attention gated networks: Learning to leverage salient regions in medical images. Medical image analysis, 53, (pp. 197-207).
Hansen, L., Diesel, J., & Heinrich, M. P. (2018). Multi-Kernel Diffusion CNNs for Graph-Based Learning on Point Clouds. In Proceedings of the European Conference on Computer Vision Workshops (ECCVW) (pp. 1-9).
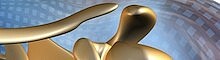
- Research
- AI und Deep Learning in Medicine
- Medical Image Processing and VR-Simulation
- Integration and Utilisation of Medical Data
- Sensor Data Analysis for Assistive Health Technologies
- Medical Image Computing and Artificial Intelligence
- Medical Data Science Lab
- Medical Deep Learning Lab
- Junior Research Group Diagnostics and Research of Movement Disorders
- Former Medical Data Engineering Lab
Contact Person
Mattias Heinrich
Professor
Gebäude MFC2, 4.OG
mattias.heinrich(at)uni-luebeck.de
+49 451 3101 5602