ARTEMIS: Artificial intelligence musculoskeletal disorders study
The ARTEMIS-Consortium hat set its goal to employ artificial intelligence and automated image processing algorithms to support radiological diagnosis and prognosis of disease risk in the field of musculoskeletal diseases. This mainly includes the assessment of the musculoskeletal system for osteoporosis, as well as differential diagnostic assessment concerning degenerative deformities. The precise objectives that are being pursued are the following:
- Opportunistically acquired CT-Scans will be interpreted automatically, in order to determine a patient individual 10-year-fracture-risk
- A system that is based on artificial intelligence will be developed to support radiologists and clinicians by pointing the user to relevant features of disease. These will be separated into osteoporotic bone fragility fo the thoracolumbar spine and the proximal femur and degenerative deformation of the lumbar spine
In collaboration with the other partners of the consortium we will contribute to developing an holistic approach to risk assessment and diagnostic assistance of spinal diseases. This approach involves radiological biomarkers, light-weight artificial intelligence models and interpretable visualisation techniques, particularly through by employing robust geometric learning techniques and attention focussed neural networks.
Thereby, we aim to improve the clinical value of the AI- support system by ensuring the interpretability through visualising the underlying decision process and activation patterns of the neural network. In order to prove the value of our methodological innovations, we will test the following research hypotheses:
- Does the integration of clinical meta-parameter improve the learning process of AI-models for automated extraction of disease specific features for spinal diseases in CT-Scans?
- Are light-weight AI-models capable of delivering equally accurate diagnosis and prognosis predictions of spinal diseases, while shortening training and inference time for the clinical IT setting?
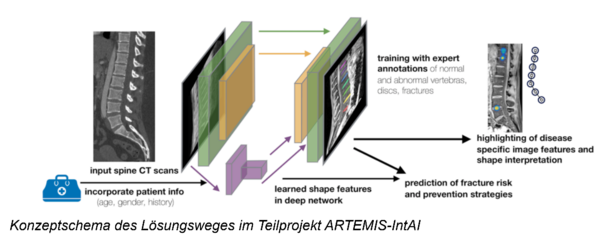
Selected Publications
MP Heinrich, O Oktay, N Bouteldja, OBELISK-Net, Fewer Layers to Solve 3D Multiorgan Segmentation with Sparse Deformable Convolutions, Medical Image Analysis,
54, 1-9 2019
J Schlemper, O Oktay, M Schaap, MP Heinrich, B Kainz, B Glocker, D Rueckert:
"Attention gated networks: Learning to leverage salient regions in medical images".
Medical image analysis, 53, 197-207. 2019
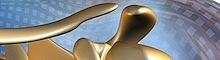
- Research
- AI und Deep Learning in Medicine
- Medical Image Processing and VR-Simulation
- Integration and Utilisation of Medical Data
- Sensor Data Analysis for Assistive Health Technologies
- Medical Image Computing and Artificial Intelligence
- Medical Data Science Lab
- Medical Deep Learning Lab
- Medical Data Engineering Lab
- Junior Research Group Diagnostics and Research of Movement Disorders
Contact Person
Mattias Heinrich
Professor
Gebäude MFC2, 4.OG
mattias.heinrich(at)uni-luebeck.de
+49 451 3101 5602