Towards Realtime MR-guided Motion Compensation using Model-based Registration without Fiducial Markers
Physiological patient motion is an important problem in accurate dose delivering during radiotherapy. Accurate and realtime motion compensation based on image-guidance could be realised in a combined MR-radiotherapy treatment setup. The objective of this project is to develop algorithms that can estimate intra-fraction motion reliably without implanted fiducial markers and improve on state-of-the-art techniques in terms of accuracy and computational speed.
In contrast to previous work, which predominantly used template matching to achieve realtime speed, we propose to incorporate prior knowledge as well as patient-specific information of plausible deformations during motion estimation. Superior motion estimation, especially for peripheral organs of risk, will be achieved using these models. To account for motion variability, MR images with high temporal resolution acquired during a short setup phase under free breathing could be incorporated for a patient-specific training. Building upon previous work, a motion-model based on principal component analysis or a Bayesian framework can be robustly trained using highly efficient deformable registration. Multiple distributed keypoints at discriminative geometric locations will be extracted automatically using machine learning techniques to avoid the need for invasive implantation of fiducial markers. Robust and accurate realtime motion estimation will be performed within a computationally efficient optimisation framework that incorporates the training model for plausible regularised motion estimation and avoids tracking errors by sampling a large space of potential motion vectors. The algorithms will be validated on retrospective clinical 4D MRI scans using manually annotated landmarks to demonstrate its suitability and advances over state-of-the-art methods.
The project is funded by Deutsche Forschungsgemeinschaft (DFG) (HE 7364/1-1).
Selected Publications
- Wilms M., Ha I.Y., Handels H., Heinrich M.P.
Model-based Regularisation for Respiratory Motion Estimation with Sparse Features in Image-guided Interventions
MICCAI 2016 - Ha I.Y., Wilms M., Heinrich M.P.
Multi-object segmentation in chest X-ray using cascaded regression ferns
BVM 2017 - Heinrich M.P., Papiez B.W., Schnabel J., Handels H.
Non-Parametric Discrete Registration with Convex Optimisation
WBIR 2014
Project Team
M.Sc. In Young Ha
M.Sc. Matthias Wilms
Jun.-Prof. Dr. Mattias P. Heinrich
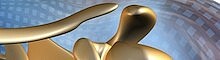
- Research
- AI und Deep Learning in Medicine
- Medical Image Processing and VR-Simulation
- Integration and Utilisation of Medical Data
- Sensor Data Analysis for Assistive Health Technologies
- Medical Image Computing and Artificial Intelligence
- Medical Data Science Lab
- Medical Deep Learning Lab
- Junior Research Group Diagnostics and Research of Movement Disorders
- Former Medical Data Engineering Lab