Learning Contrast-invariant Contextual Local Descriptors and Similarity Metrics for Multi-modal Image Registration
Deformable image registration is a key component for clinical imaging applications involving multi-modal image fusion, estimation of local deformations and image-guided interventions. A particular challenge for establishing correspondences between scans from different modalities: magnetic resonance imaging (MRI), computer tomography (CT) or ultrasound, is the definition of image similarity. Relying directly on intensity differences is not sufficient for most clinical images, which exhibit non-uniform changes in contrast, image noise, intensity distortions, artefacts, and globally non-linear intensity relations (for different modalities).
In this project algorithms with increased robustness for medical image registration will be developed. We will improve on current state-of-the-art similarity measures by combining a larger number of versatile image features using simple local patch or histogram distances. Contrast-invariance and strong discrimination between corresponding and non-matching regions will be reached by capturing contextual information through pair-wise comparisons within an extended spatial neighbourhood of each voxel. Recent advances in machine learning will be used to learn problem-specific binary descriptors in a semi-supervised manner that can improve upon hand-crafted features by including a priori knowledge. Metric learning and higher-order mutual information will be employed for finding mappings between feature vectors across scans in order to reveal new relations among feature dimensions. Employing binary descriptors and sparse feature selection will improve computational efficiency (because it enables the use of the Hamming distance), while maintaining the robustness of the proposed methods.
A deeper understanding of models for image similarity will be reached during the course of this project. The development of new methods for currently challenging (multi-modal) medical image registration problems will open new perspectives of computer-aided applications in clinical practice, including multi-modal diagnosis, modality synthesis, and image-guided interventions or radiotherapy.
The project is funded by Deutsche Forschungsgemeinschaft (DFG) (HE 7364/2-1).
Selected Publications
- Heinrich M.P., Blendowski M.
Multi-Organ Segmentation using Vantage Point Forests and Binary Context Features
MICCAI 2016 - Blendowski M., Heinrich M.P.
Kombination binärer Kontextfeatures mit Vantage Point Forests zur Multi-Organ-Segmentierung
BVM 2017 - Heinrich M.P., Jenkinson M., Bhushan M., Matin T., Gleeson F.V., Brady S.M., Schnabel J.A.
MIND: modality independent neighbourhood descriptor for multi-modal deformable registration.
Medical image analysis 2012 - Heinrich M.P., Jenkinson M., Papiez B.W., Brady S.M., Schnabel J.A.
Towards realtime multimodal fusion for image-guided interventions using self-similarities
MICCAI 2013
Project Team
M.Sc. Max Blendowski
Jun.-Prof. Dr. Mattias P. Heinrich
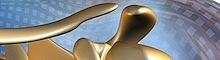
- Research
- AI und Deep Learning in Medicine
- Medical Image Processing and VR-Simulation
- Integration and Utilisation of Medical Data
- Sensor Data Analysis for Assistive Health Technologies
- Medical Image Computing and Artificial Intelligence
- Medical Data Science Lab
- Medical Deep Learning Lab
- Medical Data Engineering Lab
- Junior Research Group Diagnostics and Research of Movement Disorders
Contact person
Mattias Heinrich
Professor
Gebäude MFC2, 4th floor
mattias.heinrich(at)uni-luebeck.de
+49 451 3101 5602