Probabilistic Statistical Shape and Appearance Models for Robust Multi-Object Segmentation in Medical Image Data
The objective of the project is to develop a model based method for automatic 3D segmentation of multiple anatomic objects in medical image volumes. The knowledge based segmentation of organs will open up new possibilities in the quantitative radiology, radiation therapy and operation planning. The main focus of this DFG project is the improvement and extension of the statistical shape models with probabilistic point correspondences that had been developed in a previous project by our research group. The probabilistic statistical shape model that already holds the information about the shape of an organ will be extended to a probabilistic shape and appearance model that additionally contains knowledge about the local appearance of organs and the global neighborhood relations between them. Furthermore the probabilistic shape and appearance models will be integrated in an advanced level set segmentation approach to enable a robust and flexible multi-object segmentation of organ ensembles in 3D image volumes.
This projekt is supported by the German Research Foundation (DFG: HA 2355/7-2).
Publications
- Hufnagel H., Ehrhardt J., Pennec X., Ayache N., Handels H., Coupled Level Set Segmentation Using a Point-Based Statistical Shape Model Relying on Correspondence Probabilities, In: Dawant B. M., Haynor D.R. (eds.), Image Processing, SPIE Medical Imaging 2010, Orlando, Vol. 7623, 1B1-1B8, 2010
- Hufnagel H., Ehrhardt J., Pennec X., Ayache N., Handels H., Computation of a Probabilistic Statistical Shape Model in a Maximum-a-posteriori Framework, Methods of Information in Medicine, 48, 4, 314-319, 2009
- Hufnagel H., Ehrhardt J., Pennec X., Ayache N., Handels H., Level Set Segmentation Using a Point-Based Statistical Shape Model Relying on Correspondence Probabilities, Workshop Probabilistic Models for Medical Image Analysis, PMMIA 09, Medical Image Computing and Computer-Assisted Intervention - MICCAI 2009, London, United Kingdom, 34-44, 2009
- Hufnagel H., Pennec X., Ehrhardt J., Ayache N., Handels H., Generation of Statistical Shape Models with Probabilistic Point Correspondences and Expectation Maximization – Iterative Closest Point Algorithm, International Journal of Computer Assisted Radiology and Surgery, 2, 5, 265-273, 2008
Project Team
M.Sc. Julia Krüger
Dr. Jan Ehrhardt
Prof. Dr. Heinz Handels
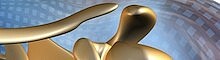
- Research
- AI und Deep Learning in Medicine
- Medical Image Processing and VR-Simulation
- Integration and Utilisation of Medical Data
- Sensor Data Analysis for Assistive Health Technologies
- Medical Image Computing and Artificial Intelligence
- Medical Data Science Lab
- Medical Deep Learning Lab
- Junior Research Group Diagnostics and Research of Movement Disorders
- Former Medical Data Engineering Lab