LABEL: Automatic labelling of anatomies in large-scale medical image datasets using self-supervised multimodal learning (DFG)
The analysis of medical image data has made a great progress in the past thanks to the novel deep learning techniques. However, large datasets that represent a comprehensive cross-section of the population and allow reliable detection of normal anatomy and abnormalities using machine learning are still missing for a successful establishment in healthcare.
The goal of the LABEL project is the development of robust and efficient algorithms for the automatic segmentation of the large-scale comprehensive population study - NAKO - that contains more than 30,000 whole-body MRI scans.
The central focus in the LABEL project will be set on enhancement of methods for the automatic segmentation of MR images with as few annotated examples as possible. For this purpose techniques of self-supervised pre-training, multimodal transfer learning and learning based image registration will be combined and extended into novel algorithms.
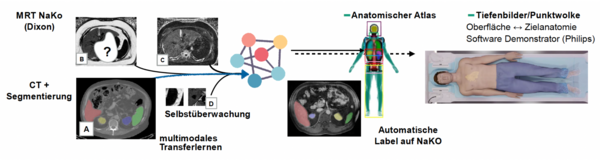
Farther, a geometric 3D atlas of internal anatomies will be created based on automatically labeled large-scale NAKO dataset. This atlas in conjunction with techniques of geometric deep learning will enable development of algorithms for precise localization of internal organs from the body surfaces. Integration of the automated anatomies localization into the MRI acquisition workflow will shorten the entire scan process and ensure significant economic advantage.
In the framework of LABEL project three partners - University of Luebeck (IMI), Fraunhofer Institute (MEVIS) and Phillips - will collaborate on development of novel deep learning methods.
The project is funded by the DFG with 426.360€
Selected Publications
Blendowski, M., Nickisch, H., Heinrich, M.P. How to Learn from Unlabeled Volume Data: Self-supervised 3D Context Feature Learning. Medical Image Computing and Computer Assisted Intervention - MICCAI, 2019.
Blendowski, M., Bouteldja, N., Heinrich, M.P. Multimodal 3D medical image registration guided by shape encoder–decoder networks. Int J CARS 15, 269–276, 2020.
Hansen L, Heinrich M.P. GraphRegNet: Deep Graph Regularisation Networks on Sparse Keypoints for Dense Registration of 3D Lung CTs. IEEE Trans Med Imaging, 2021.
Project Team
![[Translate to english:] P70 LABEL [Translate to english:] P70 LABEL](/fileadmin/_processed_/e/c/csm_csm_label_mi_master_01_19a959dd26_0edd5d1b1c.jpg)
- Research
- AI und Deep Learning in Medicine
- Medical Image Processing and VR-Simulation
- Integration and Utilisation of Medical Data
- Sensor Data Analysis for Assistive Health Technologies
- Medical Image Computing and Artificial Intelligence
- Medical Data Science Lab
- Medical Deep Learning Lab
- Junior Research Group Diagnostics and Research of Movement Disorders
- Former Medical Data Engineering Lab